NVIDIA Pairs Cosmos And Omniverse To Advance Physical AI For Robotics And Cars
To say that NVIDIA is quite an AI powerhouse is a gargantuan understatement. Most enthusiasts know the company for its GeForce graphics cards, but machine learning has driven NVIDIA's profits for many consecutive quarters at this point. The company's Tensor cores and GPU architecture drives all kinds of artificial intelligence initiatives. And speaking of driving, the company tonight at CES 2025 announced a big boost for its DRIVE autonomous vehicle platform: generative physical AI. Welcome to Cosmos Physical Generative AI.
Generating Training Data With Cosmos
Cosmos itself isn't new; it's NVIDIA's platform for accelerating AI development, and it's integrated into all kinds of frameworks like TensorRT, the CUDA Deep Neural Network (CuDNN) library, the company's graphics drivers, and so on. It exists at just about every level of the software development stack to ensure high-performance, optimized routines that are custom-built for NVIDIA's AI hardware. What's new is the first wave of physics-based simulation and synthetic data generation. It's a high-powered way to train models that will do things in the real world, like drive cars and automate warehouses.NVIDIA has created a library of ready-to-go open diffusion and autoregressive transformer models to generate video using proper physics. That last part is really, really important when it comes to training models so that cars can drive themselves and not mangle pedestrians, or warehouse robots can drive forklifts and not run into other vehicles or take out walls. Being able to generate video for models to be trained on and knowing that the physics are based in the real world means you're not poisoning a model before it ever gets off the ground.
Cosmos models have been trained on more 9 quadrillion (that's a 9 followed by twelve zeroes) tokens from over 20 million hours of video encompassing human interactions, environmental data, driving data, and more. NVIDIA is releasing three flavors of its different Cosmos models: Nano, Super, and Ultra. Nano is for edge deployment on storage-sensitive and low-latency devices. Super models (the AI variety, not Heidi Klum) are meant for building high-quality reference models. And finally, Ultra is for the highest fidelity and are, by NVIDIA's recommendation, best for distilling custom AI models.
NVIDIA has released the foundational models under the company's Open Model License Agreement. The company says Cosmos models were developed with NVIDIA's Trustworthy AI principles, including non-discrimination, privacy, safety, and transparency. The models include Cosmos Guardrails, a suite of models that work to mitigate harmful text and image inputs during preprocessing and training, and generates videos for review during post processing. Like the models themselves, developers are free to add further safety enhancements, as well.
Convert Training Data to Training Video With Omniverse
NVIDIA reckons that when paired with its Omniverse Platform -- used for three-dimensional scene descriptions -- Cosmos open models can create entire worlds in video form, on which new models can be trained, all without having to go through a manual process of setting up and recording scenarios.This sums up the definition of Physical Generative AI -- machine learning trained on video from the real world to generate more video to train other models. Using a combination of real video and generative AI, Cosmos models use real-world physics and real-world 3D objects to make valid training data suitable for AI in the physical world, which is where NVIDIA's DRIVE and other robotics platforms come in.
It's important to recognize that NVIDIA is not claiming that generative training video can in any way entirely replace real-world video. The generated data augments what's been collected from meatspace to add to the available data to train physical AIs. Self-training AI isn't here just yet, and it's still kind of hard to imagine a world in which it exists.
Omniverse is also gaining a new generative feature: Sensor RTX. The larger a model, the more diverse the training data has to be, and it needs to be fine-tuned for validation. Collecting that data can be dangerous, especially when trying to simulate something that it, itself, dangerous. To make up for that, Omniverse Cloud will gain Sensor RTX APIs that enable physically-accurate sensor information to generate datasets at scale. This is an early-access feature, which is in part why it's limited to just cloud GPUs at the moment, but expect to hear more about that in the coming months.
NVIDIA DRIVE Gets Next-Gen Design Wins
The company also announced tonight that it has earned some big design wins from a variety of automotive manufacturers. Toyota, Aurora, and Continental have all signed on to build vehicle AI on NVIDIA's platform. That adds to a growing list of manufacturers that NVIDIA estimates will grow to a $5B chunk of the company's business in its 2026 fiscal year.Toyota is building its next generation of consumer vehicles with NVIDIA's DRIVE AGX Orin SoC running the DriveOS operating system. The company's vehicles will be built on the DRIVE Hyperion platform, which just received certification in multiple industry safety tests by TÜV SÜD and TÜV Reinland.
Aurora and Continental, meanwhile, have developed a long-term strategic partnership to deploy driverless trucks at scale. For instance, the Aurora Driver is an SAE Level 4 autonomous driving system that Continental will manufacture for deployment in 2027.
Unsurprisingly, both Cosmos and Sensor RTX will play a very large role in the advancement of NVIDIA DRIVE and DriveOS. The company needs to continue to collect data on edge cases and unsafe conditions without putting people in jeopardy, and Cosmos will be able to generate that data using realistic sensor simulations from Sensor RTX's APIs. This, the company says, will continue to accelerate the rate at which it's able to provide safer autonomous vehicles, getting people and goods where they need to go safely.
NVIDIA Accelerates Humanoid Robotics With Isaac GR00T
Finally, NVIDIA's Isaac is the company's robotics development platform, and the newly announced GR00T Blueprint will accelerate it with synthetic motion data. This is used to train humanoid robots with imitation learning, which is exactly what it sounds like. The robot imitates what its sees an expert human doing to learn a new skill. The GR00T Blueprint can generate synthetic datasets from a small number of human demonstrations. Generative physical AI is a theme, and this is another expression of the concept.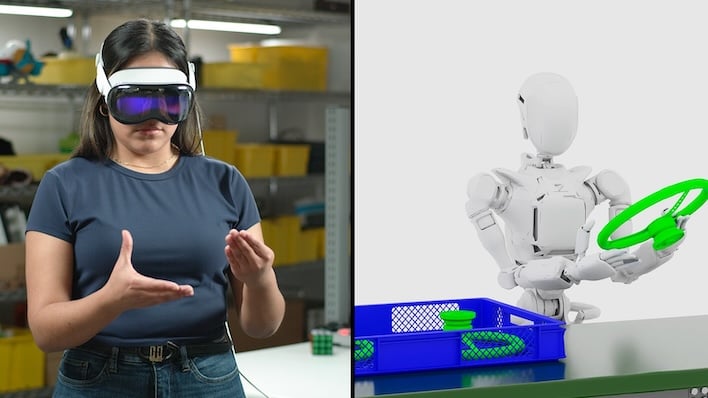
As we mentioned several months back, NVIDIA is bringing its AI smarts to the Apple's Vision Pro to capture human actions in a digital twin -- in this case, a replica of the human motion. The human actions are imitated by a robot in a simulation, and those recordings are a "record of truth" of sorts. GR00T-Gen exponentially expands the dataset through its use of 3D upscaling and domain randomization. The dataset can be used to help train the robots to do new tasks with less effort required up front to generate all the video.