Computer Scientists Claim To Have Found A Foolproof Way Of Detecting Deepfake Videos
In the case of a traditional video or image deepfake, an algorithm starts with a video or image one person, then swaps the person’s face for the face of someone else. However, instead of swapping faces, deepfake technology can change a person’s facial expressions. Both of these capabilities have the potential for nefarious applications. Deepfake technology could be used to doctor or create a video of someone acting or speaking in an unscrupulous manner for purposes of character assassination motivated by revenge, political gain, or cruelty. Even the possibility of deepfakes undermines trust in video, image, or audio evidence. Some researchers have responded by developing ways to detect deepfakes by leveraging the same machine learning technology that makes them possible.
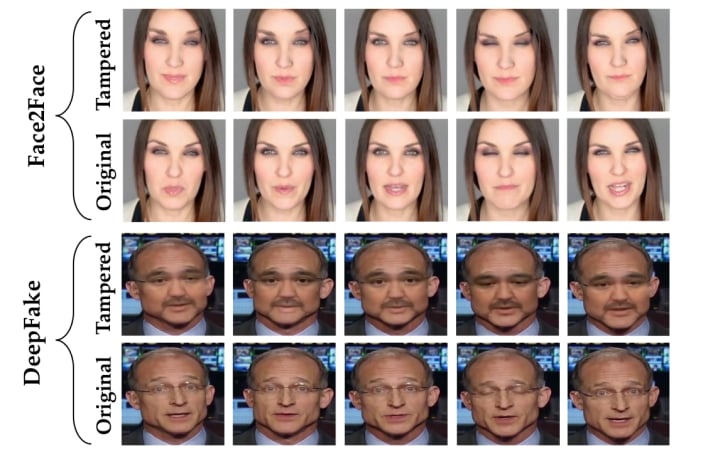
The new framework, which the computer scientists named “Expression Manipulation Detection” (EMD), first maps facial expressions, then passes that information on to an encoder-decoder that detects manipulations. The framework is able to indicate which areas of a face have been manipulated. The researchers applied their framework to the DeepFake and Face2Face datasets and were able to achieve 99% accuracy for detection of both identity and expression swaps. This paper gives us hope that automatic detection of deepfakes is a real possibility.