New Synopsys Big Data Analytics Tools To Fuel Chipmakers With AI-Driven Insights
This advancement is a significant next step for EDA tools and the larger chip industry as a whole. EDA is leveraged at just about every point of the chip design flow, including testing, simulation, and verification. It generally allows engineers to work at a layer abstracted above the individual transistors by operating with so called “cells” of logic. Libraries of these cells and their functions then become the building blocks for a chip, and EDA software helps stitch them together. Though this may be a gross oversimplification, that's the general gist. In addition, Synopsys has also regularly been infusing its EDA tools with AI to automate this process even further, with a place-and-route tool called DSO.ai or Design Space Automation AI, for example.
It has historically been a one-way process on the software side, though. Working at the bleeding-edge of silicon processes often exposes new and different restrictions, which have to be manually fed back into the process as updated design rules. These could include factors like transistor gate pitch, signal timing, spacing, and the list goes on. ICs are typically designed with multiple layers of increasing complexity. The rules get stricter as the features of a given layer shrink as lithography processes have difficulty cleanly outlining patterns. There's probably a million and one ways to improve silicon designs but finding them early is a significant challenge.
Leveraging AI To Drive Silicon Design Insights
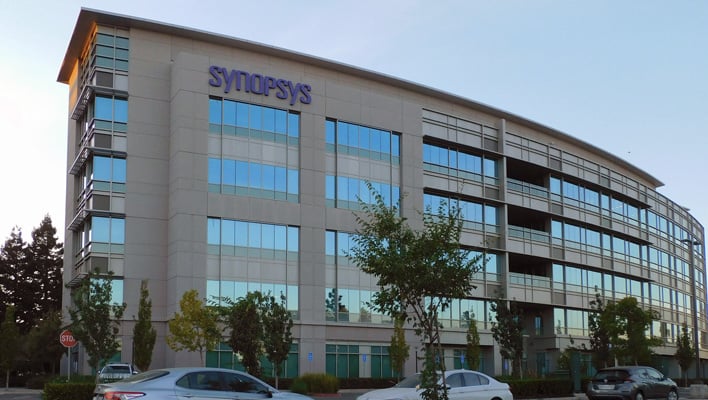
(Via: Chang Architecture)
“As IC complexity grows and market windows shrink, the semiconductor industry is increasingly adopting artificial intelligence technologies to enhance the quality of results (QoR), speed verification and testing, improve fab yield, and boost productivity across multiple domains spanning the entire IC design flow,” said Sanjay Bali, vice president of Strategy and Product Management for the EDA Group at Synopsys. “With the new data analytics capabilities within the Synopsys.ai EDA suite, companies can now aggregate and leverage data across every layer of the EDA stack from architecture exploration, design, test, and manufacturing to drive improvements in PPA, yield, and engineering productivity.”
The Synopsys EDA Data Analytics solutions are claimed to be the industry-first for providing AI-driven insights and optimizations. It and is divided into three packages. Synopsys Design.da leverages all the unstructured, multi-domain data generated to provide chip designers with “actionable design insights” that can help improve the holy trinity of power, performance, and area (PPA). Meanwhile, Synopsys Fab.da can extract process control data from fabs to improve operational efficiencies leading to better product quality and fab yield, while Synopsys Silicon.da acts on silicon monitoring, diagnostic, and production test data to improve quality, yield, and important silicon metrics like power and performance. These factors can feed back into the design process sooner, hopefully catching potential issues in the mask, fabrication, and test processes before they happen.
“The volume of data generated during chip manufacturing and testing is massive, making big data tools essential to analyze and extract meaningful conclusions from these data sets,” said Dr. Greg Bazan, senior principal engineer at Marvell. “The Synopsys chip data analytics tool has been vital to improve the efficiency and quality of our manufacturing process. We look forward to experiencing how the benefits of Synopsys’ next-generation analytics tool can further improve our KPIs and reduce manufacturing and test costs for our next-generation products.”
Synopsys says its EDA Data Analytics solution is available now and ready for customers. We look forward to seeing what these machine-learning-enhanced analytics can help chipmakers achieve. Beyond improving designs and efficiency, the ramp from early silicon samples to high volume manufacturing is a particularly vulnerable time for setbacks to occur, so having these tools could potentially help manufacturers navigate past roadblocks before they become an issue. The result is then a faster time to market, better margins, and potentially a lower cost for us as the consumers.