Google's DeepMind Agent57 AI Masters Classic Atari Games Like Pitfall And Solaris
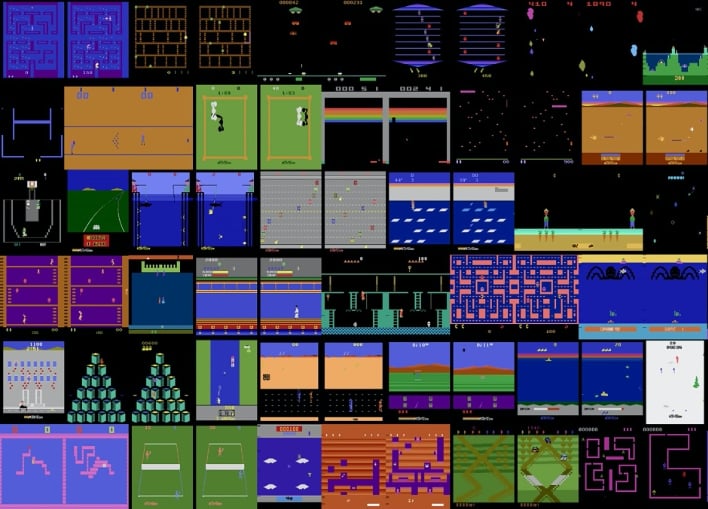
The DeepMind team often looks to existing games to help improve its AI routines, and it explains that they provide “excellent testing ground for building adaptive algorithms” that can encompass a “rich suite of tasks which players must develop sophisticated behavioral strategies to master.” More importantly, each game has its own high score which can be used as a benchmark for improved performance in the future.
A number of well-known titles were included in the grouping of 57 like Solaris, Skiing, Montezuma’s Revenge, and Pitfall. These four games in particular were chosen because previous attempts to tackle these games with AI have failed miserably at being successful. In the case of Montezuma’s Revenge and Pitfall, the aspect of exploring the game environment was the pitfall with previous attempts.
In the case of Skiing and Solaris, the DeepMind team writes:
[These games] are long-term credit assignment problems: in these games, it’s challenging to match the consequences of an agents’ actions to the rewards it receives. Agents must collect information over long time scales to get the feedback necessary to learn.
However, the DeepMind team was able to make optimizations to the Deep-Q network to improve decision-making skills and take better advantage of the reward systems (using a time horizon) of games like Solaris. They then leveraged exploration options in Pitfall, which are then funneled through an adaptive meta-controller.
In the end, Agent57 was able to learn how to play all 57 games, one at a time, retraining before moving on to the next title. This “limitation” is also inherent in DeepMind’s AlphaZero AI, and is something that the team hopes to be able to move beyond in the future to make such systems more human. "True versatility, which comes so easily to a human infant, is still far beyond AIs' reach,” the team added.